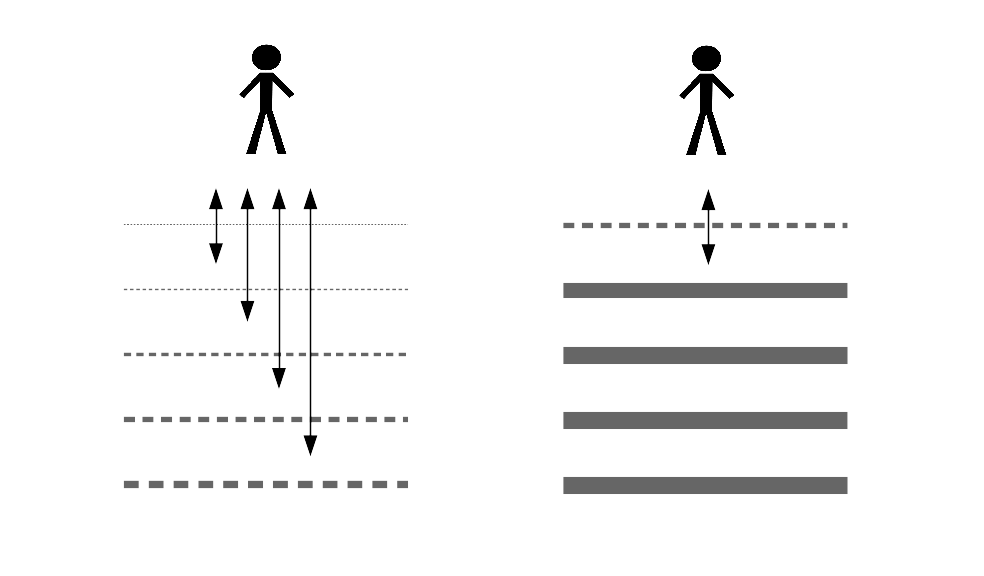
For centuries we've designed complex systems through a superposition of layers. The outer layer, the "user interface," is in direct contact with users. The inner layers correspond to subsystems that work on behalf of the users, but with which users have little or no direct interactions.
Everything is designed that way, not only software and gadgets:
- Food is a complex system in which the user interface includes package labels, supermarket shelves, and restaurant menus.
- Democracy is a complex system in which the user interface includes voting ballots, media coverage of politics, and demonstrations.
- Banking is a complex system in which the user interface includes automatic and human tellers, point of sale systems, and stock tickers.
- Healthcare is a complex system in which the user interface includes waiting rooms, nurses, doctors, and pharmacists.
- Cars are complex systems in which the user interface includes the steering wheel and the dashboard.
We use many metaphors to speak about the inner layers. We speak of what happens in the "back office" or "under the hood." We assume whatever happens there doesn't concern us as long as the job gets done. System designers assume, in turn, that most of the time users don't need to know about the inner layers. Indeed, when an inner layer is inadvertently exposed through the interface we see this as a system failure; sometimes a benign one such as a bit of dirt in supermarket vegetables, sometimes a creepy one such as a chicken head in a bag of McNuggets.
All systems require transparency to use them effectively, to recover from failure, and to build upon them.
Despite what naïve system designers may think, in most systems users often need to know about what internal layers are doing: to use a system effectively, to recover from failure, and to build upon it or customize it to their needs. For instance:
- A healthy diet requires knowing what goes into our food. A sustainable diet requires knowing where does it come from and how it is prepared.
- Democracy cannot be construed to mean blindly voting every few years, and actual progress requires understanding how legislative change works.
- Reducing the harm from the next economic crisis, requires understanding what can each of us should do to prevent it from being too deep.
- Maintaining good health and recovering from illness without bankrupting hospitals, increasingly requires relying of patients' self-care.
- Fuel economy and safe driving requires a basic understanding of how a car engine works, and what are the symptoms of possible failure.
Some of the biggest crises we're facing today are caused or aggravated by the fact that we're hiding too much information in the name of "simplicity." We're increasingly becoming separated of sub-systems that are vital for us. Complete opacity is bad design, sometimes intentionally so. Usable systems have a transparent outer layer and ways of interacting with inner layers progressively.
Power structures that predate the information age deal with opacity by creating more opacity.
The way in which this information problem is addressed, and sometimes ignored, mirrors pre-information age power structures. Indeed, they tend to encourage more opacity: instead of transparency and access to information, we see more and more layers of specialists, representatives and regulators who are supposed to represent our interests and keep us safe:
- Food production companies fight tooth and nail against any initiative to expand information to consumers. Instead, they encourage industry-led committees that determine what is good for us.
- Instead of implementing transparency by default, most governments implement transparency on request, often behind a bureaucratic maze.
- Banking oversight as implemented now requires us to blindly trust on the same people who repeatedly failed to prevent banking crises.
- The only information we get about pharmaceuticals are advertisings encouraging us to use the newest medications.
- Car diagnostics are hidden through proprietary systems that make (self-)maintenance impossible or artificially more expensive.
Professionals that create computing and information processing systems have our share of responsibility on this. Not only we design big parts of these systems, we create the wrong metaphors than shape the industries and the expectations of users.
We're encouraging users to believe that ignorance is a good thing.
Most notably, we're encouraging people to believe that ignorance is a good thing. Systems where users are "taken care of" and "don't need to worry about anything" should arise suspicion, not praise. As the world becomes more complex and people have a proportionally vanishing understanding of what happens around them, we should enable and encourage exploration.
A possible framework to achieve this is what Jonathan Zittrain calls generative systems, but there is much more to it. The starting point, in my opinion, is to realize that an entire generation is actively being prevented from understanding the systems around them. This is a huge step backwards. People learn about the systems around them by using them: let's encourage and enable that learning.